Assistant Professor, The University of Alabama
** This site has not been updated regularly. For most recent updates, please visit my Personal Site at the University. Thank you!! **
Dr. Liu's primary research goal is to generate knowledge that stimulates applications of new and innovative strategies, technologies, and planning processes in transportation systems. His research focuses on various types of innovations related to intelligent transportation systems (ITS), connected and automated vehicles (CAVs), traffic safety and operation, urban/transportation planning, and sustainable transportation. He exploits large-scale data (from both conventional and emerging sources) and construct microscopic simulations (on agent-based modeling platforms) to understand the roles of travelers, vehicles, infrastructures and policies in transportation systems and to develop solutions to transportation challenges related to planning, operation, safety and environment.
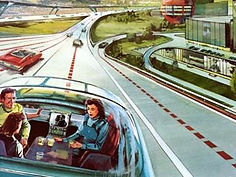
Dr. Liu's has touched both the traditional transportation topics and emerging topics related to transportation.
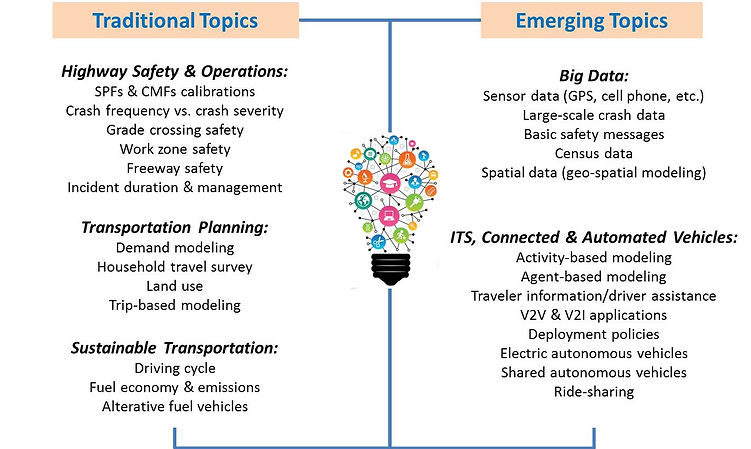
Below are selected topics that Dr. Liu has been working on:
Deloployment of CAVs at Higher Levels of Automation - Shared Autonomous Vehicles (SAVs)
When fully automated vehicles are ready for public use, shared autonomous vehicles (SAVs) are expected to become a major travel mode as car-sharing (of human driver vehicles) is aggressively happening now in many cities. SAVs can bring expensive transport technologies to the masses well before individuals may be able to afford their own autonomous vehicle. SAVs reduce car-sharing costs by bringing empty driverless vehicles directly to the user and dropping off passengers at their requested destinations, without worrying about parking (or, presumably, refueling). This research delivers a large-scale agent-based simulation of deploying SAVs in a metropolitan area. The simulation is an activity-based travel demand model capturing dis-aggregated travel patterns, as opposed to aggregated travel modeling in conventional trip-based models.
The simulation answers an important deployment question- how many SAVs are needed in different scenarios to avoid long wait times? The take-way finding is that one SAV can meet up to 7 persons’ travel requests. However, owing to SAV’s self-driving while empty of passengers, the 10 ~ 15% increased vehicle miles traveled (VMT) may result in more congested traffic, additional energy use and emissions. Opportunities (e.g., ride-sharing, tight headways and traffic smoothing) for SAVs are also discussed in the research.
Therefore, ride-sharing, tight headways, traffic smoothing, and other opportunities for SAVs may be considered to help counteract some of these VMT increases, in terms of congestion and emissions. The work provides guideposts for private and public sector stakeholders who seek to implement SAV systems, and transportation planners and policy (and decision-) makers who must plan for arrivals of this new technology on road.
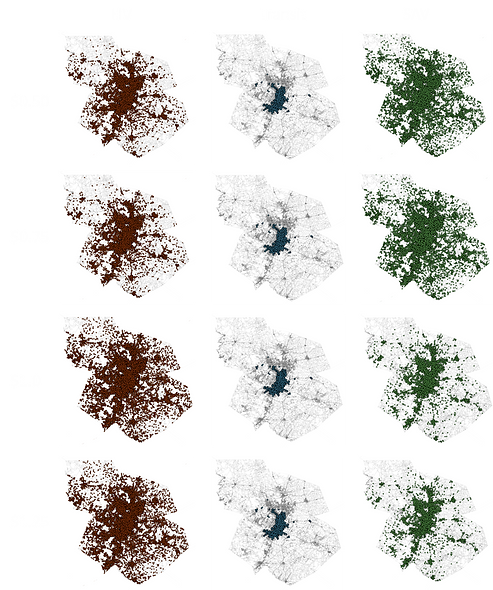
Development of CAVs at Lower Levels of Automation - CAV Safety Application Based on Historical CAV Driving Data
-
Connected and automated vehicles (CAVs) are already part of the surface transportation system and their market penetrations are likely to increase in the future. To operate a CAV safely, information needed includes static information (high-resolution navigation maps) and real-time from various sensors, some of which exchange information with other vehicles or roadside units. High resolution navigation maps can integrate historical driving volatility data to help CAVs and drivers (operating at low levels of automation) make more informed proactive choices. Specifically, this study proposes to add historical driving data in the navigation maps.
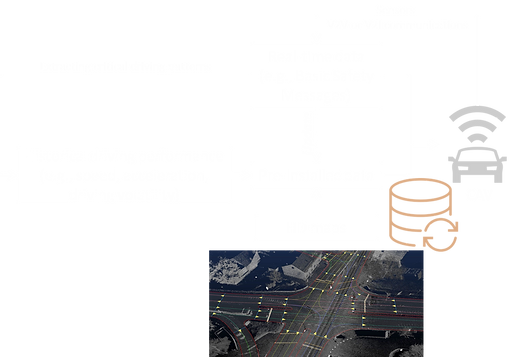
Driving data offer insights about the historical decisions made by drivers at locations along a route, e.g., where drivers often make sharp turns, accelerate or decelerate hard. Pre-installing historical driving decisions will “warn” CAV about potential hazards and help drivers make more informed speed and routing decisions. This study introduces the concept of location-based driving volatility as a key to unlocking the potential of CAVs to improve safety. Location-based volatility is a measure of historical driving performance, defined as the percentage of maneuvers that are extreme for the segment or intersection. For demonstration, real-world high-frequency geo-referenced data are visualized in space and modeled. The data were collected in a connected vehicle safety pilot program in Ann Arbor, Michigan. The measured location-based volatility was found to be significantly related to the historical safety outcomes in this region. Crashes were likely to occur at or near a location with greater driving volatility. Therefore, location-based driving volatility can serve as valuable piece of information to be added into the navigation maps for CAVs, helping them better navigate volatile hot-spots.
Location-Based Driving Volatility
-
A concept of measuring the variability of driving performance.
-
Capturing extreme maneuvers at a specific location.
-
Extreme maneuvers are identified by comparisons with the majority maneuvers (representing regional performance).
-
It requires large data to define thresholds for the majority.
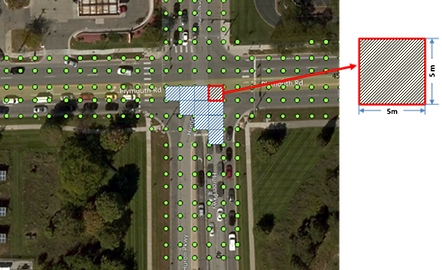
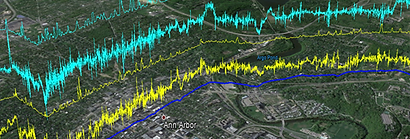
Advanced Modeling of Traffic Crashes - Geospatial & Temporal Modeling
Motivated by the spatial and temporal nature of traffic crashes, Dr. Liu has developed a variety of models to disentangle the spatial temporal patterns of crashes. Approaches include Geographically Weighted Regression (Linear, Binary Logit, Multinomial Logit, Ordinal Logit, Poisson, Negative Binomial, etc.) and Integrated Spatio-Temporal Model.

